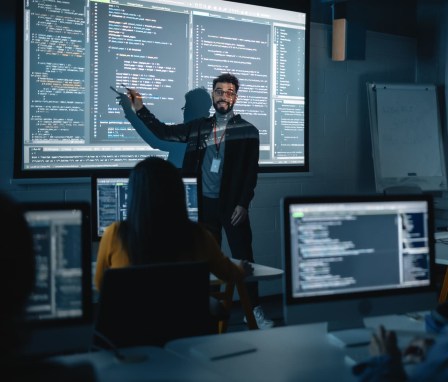
Everyone either knows a lot about artificial intelligence (AI) or very little. For fun, let's do a pop quiz.
What is AI? You get an A+ if you know it's a computer capable of human thought processes. It's much more complex than that, but let's keep it simple for now.
Now let's see if you can answer the three big questions:
- How does it work?
- How can we use it?
- What will it improve next?
If you don't have the answers but are curious to know more, then an artificial intelligence course may be the solution.
AI courses teach the many ways we make computers perform and automate tasks like humans. They're available at beginner, intermediate, and advanced levels and designed by colleges, universities, and course providers. Algebra experience is often all you need to start.
Plus, it's a good time to learn about AI. The World Economic Forum predicts AI will create 97 million new jobs by 2025. AI and machine learning specialists are the fastest-growing jobs in the world in 2023. AI courses can be useful for professionals interested in upskilling.
Here is what you learn in an AI course, including the topics you're likely to cover at each level.
Popular Online Programs
Learn about start dates, transferring credits, availability of financial aid, and more by contacting the universities below.
Beginner-Level AI Courses
Example: Introduction to AI Technology (Microsoft)
Beginner classes are an introduction to AI. You learn how computers can mimic human cognition. You become familiar with techniques like deep learning and neural networks. You may learn about the history of AI, debate ethical issues, and practice training algorithms.
Beginner classes in AI are often designed for those with little or no background in the subject. Computer science or programming experience is not always a requirement. However, instructors might expect you to have basic knowledge of discrete math, probability, and linear algebra.
A beginner AI course covers various fundamentals:
- Machine learning is a subcategory of AI that uses algorithms to allow computers to learn and make increasingly better decisions without human interference.
- Neural networks are computational models replicating the role of neurons in the human brain, with data passing through a network of interconnected artificial neurons.
- Computer vision is a computer's ability to perceive the world, including images and videos.
Intermediate-Level AI Courses
Example: Natural Language Processing Specialization (Coursera)
Intermediate-level artificial intelligence courses build on fundamental concepts. For example, you may learn about deep generative models, a branch of deep learning. Or it may be advanced computer vision techniques like objective detection and image segmentation.
Other intermediate AI courses shift the focus from conceptual to practical, involving projects and real-life scenarios. It might be a hands-on experience where you build a scale-model self-driving car. Or a program to help you find ways to integrate AI into business workflows.
Intermediate-level AI topics include:
- Deep learning is a subfield of machine learning and a more complex version of neural networks, with multiple neural layers and higher levels of pattern recognition.
- Generative models are machine learning algorithms that use real-world content like images, audio and video files to create new content, such as AI art.
- Neural machine translation is a subfield of natural language processing capable of solving difficult translation problems with end-to-end learning.
Advanced-Level AI Courses
Example: Decision Making Under Uncertainty (University of Georgia)
Advanced-level AI courses are likely to be the most rigorous, practical, or thought-provoking. They may require the heaviest workload or involve the most challenging material. These courses, therefore, require strong foundational knowledge.
Some advanced AI courses may focus on current research trends or recent breakthroughs. Others may lean more into theoretical concepts and emerging areas in AI, which represent a new frontier of innovation.
Examples of advanced AI topics include:
- Agent environment is a concept that describes various interactions between an AI system (the agent) and its surroundings, including a fully observable and partially observable environment.
- Bayesian parameter estimation is a statistical model that can make predictions about future observables.
- Generative Adversarial Networks are advanced generative models that can distinguish between real and fake data samples.
Sources
- Artificial intelligence in business: creating value with machine learning. (n.d.). Harvard University
- Ocampo, Rodolfo. (2022). AI art is everywhere right now. Even experts don't know what it will mean. The Conversation
- ETH Zurich: Self-driving cars with Duckietown. (n.d.). EdX
- The future of jobs report. (2020). The World Economic Forum
Recommended Reading
View hand-picked degree programs
Tell us what you’d like to specialize in, and discover which schools offer a degree program that can help you make an impact on the world.